Unleashing the Power of Machine Learning: A Journey into Intelligent Decision-Making
Introduction: Machine Learning (ML), a subset of artificial intelligence (AI), has emerged as a transformative force, revolutionizing industries and reshaping how we approach problem-solving. In this blog post, we’ll delve into the intricacies of Machine Learning, exploring its applications, algorithms, and the profound impact it has on shaping a smarter, data-driven future.
Understanding Machine Learning: At its core, Machine Learning is a field of study that enables computers to learn patterns and make predictions without explicit programming. It leverages algorithms and statistical models to analyze and interpret data, allowing systems to improve their performance over time. Explore the new world of rising job opportunities in the domain of Machine Learning by joining the Machine Learning Training in Hyderabad course by Analytics Path.
Types of Machine Learning:
- Supervised Learning: The algorithm is trained on a labeled dataset, learning to map input data to the corresponding output.
- Unsupervised Learning: The algorithm explores data without labeled outcomes, identifying patterns and relationships within the information.
- Reinforcement Learning: The system learns by interacting with its environment, receiving feedback in the form of rewards or penalties.
Applications of Machine Learning:
- Healthcare: ML facilitates disease diagnosis, personalized treatment plans, and drug discovery.
- Finance: Fraud detection, credit scoring, and algorithmic trading benefit from ML’s predictive capabilities.
- Retail: Recommender systems, demand forecasting, and inventory management optimize the retail experience.
- Autonomous Vehicles: ML is pivotal in enabling vehicles to perceive their surroundings, make decisions, and navigate safely.
Key Machine Learning Algorithms:
- Linear Regression: Predicts numerical values based on historical data.
- Decision Trees: Builds a tree-like model for decision-making based on input features.
- Support Vector Machines (SVM): Classifies data by finding an optimal hyperplane in a high-dimensional space.
- Neural Networks: Mimics the human brain’s structure, excelling in complex pattern recognition tasks.
Challenges and Ethical Considerations: While ML brings immense possibilities, challenges include data privacy concerns, algorithm bias, and the ethical implications of automated decision-making. Striking a balance between innovation and responsibility is crucial for the continued ethical development of ML.
Future Trends: The future of Machine Learning holds promises of advancements in Explainable AI, Federated Learning, and the integration of ML with edge computing. As technology evolves, ML will continue to be a driving force behind innovation and automation.
Conclusion: Machine Learning is not just a technological evolution; it’s a paradigm shift that empowers businesses and individuals to harness the potential within vast datasets. As we navigate the era of smart technologies, the symbiotic relationship between humans and intelligent machines will shape a future where decisions are not just data-driven but augmented by the transformative power of Machine Learning
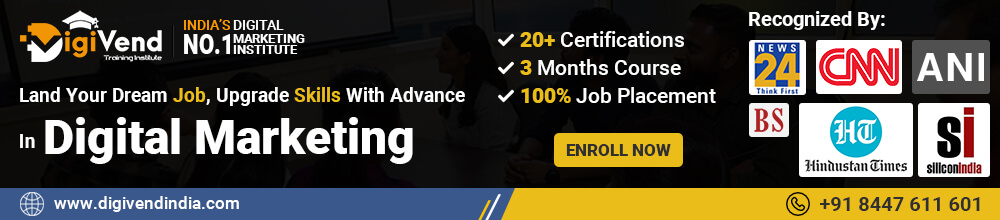