Data Analytics in Healthcare: Transforming Patient Care and Medical Outcomes
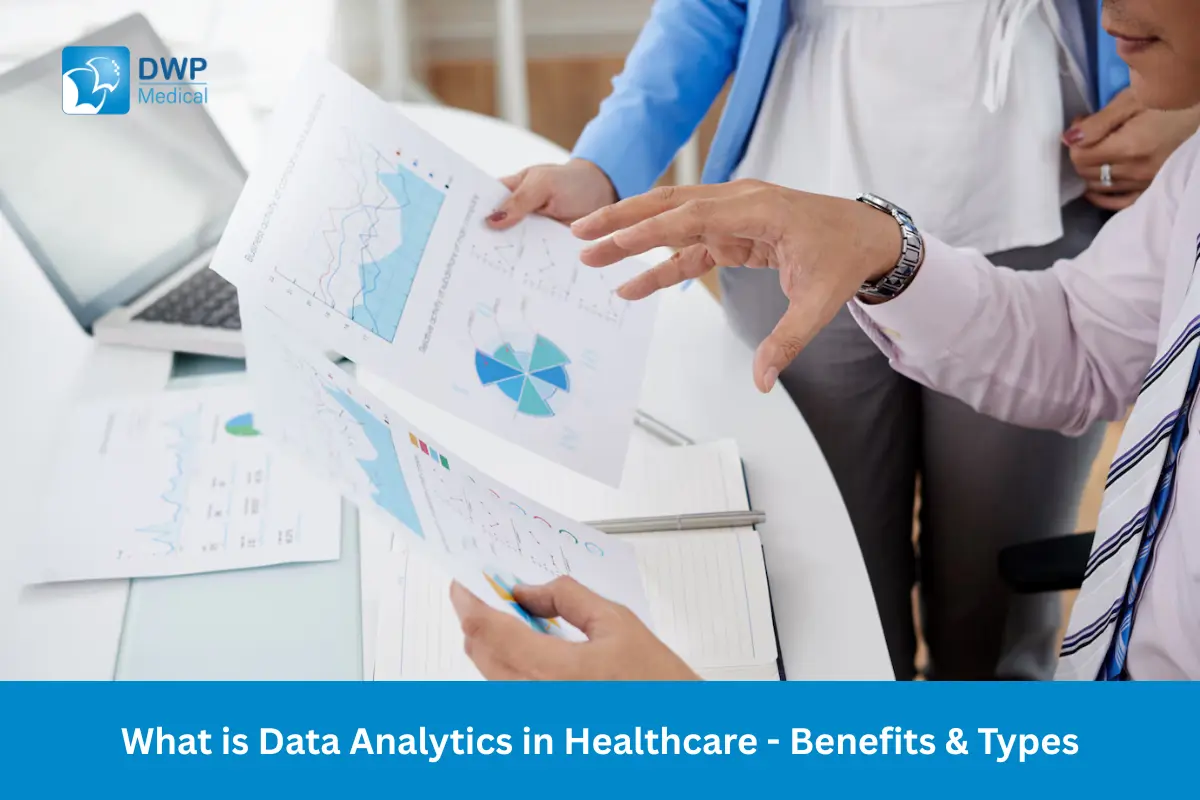
Healthcare is rapidly evolving, and Healthcare Data analytics plays a pivotal role in this transformation. But what exactly is data analytics, and how is it reshaping patient care, operational efficiency, and medical research? This article answers your most pressing questions about healthcare data analytics, offering clear insights and actionable knowledge for professionals and decision-makers alike.
What Is Data Analytics in Healthcare?
Data analytics in healthcare refers to the process of collecting, analyzing, and interpreting vast amounts of medical and operational data to improve health outcomes, streamline clinical processes, and reduce costs. It involves technologies like big data analytics, predictive analytics, and machine learning to derive meaningful patterns from complex datasets.
By turning raw data into actionable insights, healthcare providers can make faster, evidence-based decisions that enhance patient care quality and safety.
Why Is Data Analytics Important in Healthcare?
Healthcare generates enormous volumes of data daily — from patient records, lab results, imaging, to insurance claims. Data analytics in healthcare helps unlock the value hidden within these datasets by:
-
Improving diagnosis accuracy and treatment personalization
-
Enhancing patient monitoring and preventive care
-
Optimizing hospital operations and resource management
-
Reducing healthcare costs by identifying inefficiencies
-
Accelerating medical research and clinical trials
In short, it transforms the traditional reactive healthcare model into a proactive, data-driven approach.
How Does Data Analytics Work in Healthcare?
Data analytics in healthcare involves several key steps:
-
Data Collection: Gathering structured and unstructured data from electronic health records (EHRs), wearable devices, medical imaging, and more.
-
Data Integration: Combining diverse datasets into a unified platform for comprehensive analysis.
-
Data Cleaning: Ensuring accuracy by removing duplicates, correcting errors, and standardizing formats.
-
Data Analysis: Applying statistical tools, machine learning algorithms, and AI to identify trends, predict outcomes, and generate reports.
-
Data Visualization: Presenting findings through dashboards and graphs for easy interpretation by clinicians and administrators.
These steps enable healthcare organizations to extract valuable insights while ensuring data privacy and security.
What Are the Types of Data Analytics Used in Healthcare?
Healthcare uses four primary types of data analytics, each serving a distinct purpose:
Descriptive Analytics
Focuses on summarizing past data to understand what happened. For example, hospital readmission rates or average patient stay length.
Diagnostic Analytics
Examines data to explain why certain outcomes occurred, such as investigating causes behind patient complications.
Predictive Analytics
Uses historical data and machine learning to forecast future events, like predicting disease outbreaks or patient risk scores.
Prescriptive Analytics
Suggests actionable recommendations based on predictive insights, guiding treatment plans or resource allocation.
Together, these analytics types empower healthcare professionals to deliver evidence-based care and improve operational efficiency.
What Are the Benefits of Data Analytics in Healthcare?
The benefits of implementing data analytics in healthcare are extensive:
-
Enhanced Patient Outcomes: By identifying risk factors and treatment responses, analytics supports personalized medicine.
-
Cost Reduction: Predictive analytics can reduce hospital readmissions and avoid unnecessary procedures.
-
Operational Efficiency: Streamlining scheduling, staffing, and supply chain management through real-time data insights.
-
Improved Population Health Management: Tracking trends and disease patterns helps public health officials respond effectively.
-
Accelerated Medical Research: Analyzing large datasets speeds up drug discovery and clinical trial processes.
Healthcare providers who leverage data analytics see improved patient satisfaction, reduced errors, and better resource utilization.
What Are the Challenges of Data Analytics in Healthcare?
Despite its potential, data analytics in healthcare faces several challenges:
-
Data Privacy and Security: Healthcare data is sensitive, requiring strict compliance with regulations like HIPAA.
-
Data Quality and Integration: Inconsistent or incomplete data from different sources can limit analytics accuracy.
-
Technical Complexity: Advanced analytics requires skilled personnel and sophisticated infrastructure.
-
Cost of Implementation: Initial investment in technology and training can be high for some organizations.
-
Resistance to Change: Clinician adoption may be slow due to workflow disruptions or skepticism.
Overcoming these hurdles requires strategic planning, robust technology, and ongoing training.
How Is Predictive Analytics Used in Healthcare?
Predictive analytics in healthcare uses data, statistical algorithms, and machine learning to predict future outcomes with high accuracy. Examples include:
-
Predicting patient deterioration: Real-time monitoring systems alert clinicians to signs of sepsis or cardiac arrest.
-
Forecasting disease outbreaks: Public health agencies track infection trends to prepare responses.
-
Identifying high-risk patients: Helps prioritize care for chronic disease management.
-
Optimizing hospital bed management: Predictive models estimate admission rates for better capacity planning.
This proactive approach reduces emergencies, improves care coordination, and enhances patient safety.
What Role Does Artificial Intelligence Play in Healthcare Data Analytics?
Artificial intelligence (AI) is a game-changer for healthcare data analytics. AI-powered tools analyze complex medical images, interpret natural language in clinical notes, and automate routine tasks. Key AI applications include:
-
Diagnostic support: AI algorithms help radiologists detect tumors or fractures faster.
-
Personalized treatment plans: AI models analyze genetic and clinical data to recommend therapies.
-
Virtual health assistants: Chatbots provide 24/7 patient engagement and triage.
-
Operational automation: AI streamlines billing, coding, and administrative workflows.
Integrating AI with analytics enhances accuracy, speed, and scalability of healthcare insights.
How Does Data Analytics Improve Patient Experience?
Data analytics in healthcare improves patient experience by enabling more personalized, timely, and efficient care. Examples include:
-
Tailored communication: Using patient data to send customized reminders and educational materials.
-
Remote monitoring: Wearables track vital signs, reducing hospital visits and enabling early interventions.
-
Streamlined appointments: Analytics optimizes scheduling to reduce wait times.
-
Patient feedback analysis: Natural language processing identifies service gaps from surveys and reviews.
By focusing on patient-centric data, healthcare providers foster trust and satisfaction.
What Is the Future of Data Analytics in Healthcare?
The future of data analytics in healthcare is promising with advancements like:
-
Integration of genomics data for precision medicine.
-
Real-time analytics powered by IoT and 5G connectivity.
-
Blockchain for enhanced data security and interoperability.
-
Augmented intelligence combining human expertise and AI.
-
Expanded use of social determinants of health data for holistic care.
Healthcare will increasingly rely on predictive and prescriptive analytics to deliver smarter, more cost-effective care.
How Can Healthcare Providers Start Using Data Analytics?
To begin leveraging data analytics in healthcare, providers should:
-
Assess data maturity: Evaluate current data systems and identify gaps.
-
Set clear goals: Define objectives like reducing readmissions or improving chronic disease management.
-
Invest in technology: Choose scalable analytics platforms and ensure interoperability.
-
Train staff: Build analytics expertise through education and collaboration.
-
Ensure compliance: Implement strong data governance policies.
-
Partner with experts: Collaborate with analytics vendors or consulting firms.
A phased approach ensures sustainable success and maximizes ROI.
What Are Some Real-Life Examples of Data Analytics in Healthcare?
Several healthcare organizations have successfully implemented data analytics:
-
Mayo Clinic: Uses predictive analytics to identify patients at risk of heart failure and reduce hospitalizations.
-
Kaiser Permanente: Leverages big data for population health management and preventive care programs.
-
Mount Sinai Health System: Applies AI to radiology imaging for faster cancer diagnosis.
-
Cleveland Clinic: Uses analytics dashboards to improve operational efficiency and patient flow.
These cases demonstrate how data-driven decisions translate into tangible health benefits.
Conclusion:
Data analytics in healthcare is no longer optional—it’s essential for delivering quality care and operational excellence. At DWP Medical, we provide the best service in healthcare data analytics, offering tailored solutions that unlock the power of your data. From predictive modeling to AI integration, our expert team helps healthcare providers innovate and succeed in a competitive landscape.
Partner with DWP Medical to harness advanced data analytics and transform your healthcare delivery today.
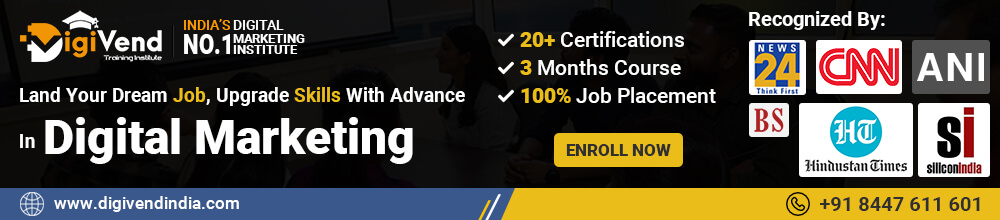